New paper in the IEEE J-STARS on wildfire monitoring using SAR data!
We are excited to share the latest paper of our team, titled “Tracking Burned Area Progression in an Unsupervised Manner Using Sentinel-1 SAR Data in Google Earth Engine,” that has been published in the IEEE Journal of Selected Topics in Applied Earth Observations and Remote Sensing (J-STARS).
It has been a fruitful international cooperation between our team members, namely Daniel Paluba, Josef Laštovička and Přemysl Štych, and teams from Italy (the EOLab at Tor Vergata University in Rome) and teams in Greece from Thessaloniki (Aristotle University of Thessaloniki) and Mitilini (University of the Aegean).
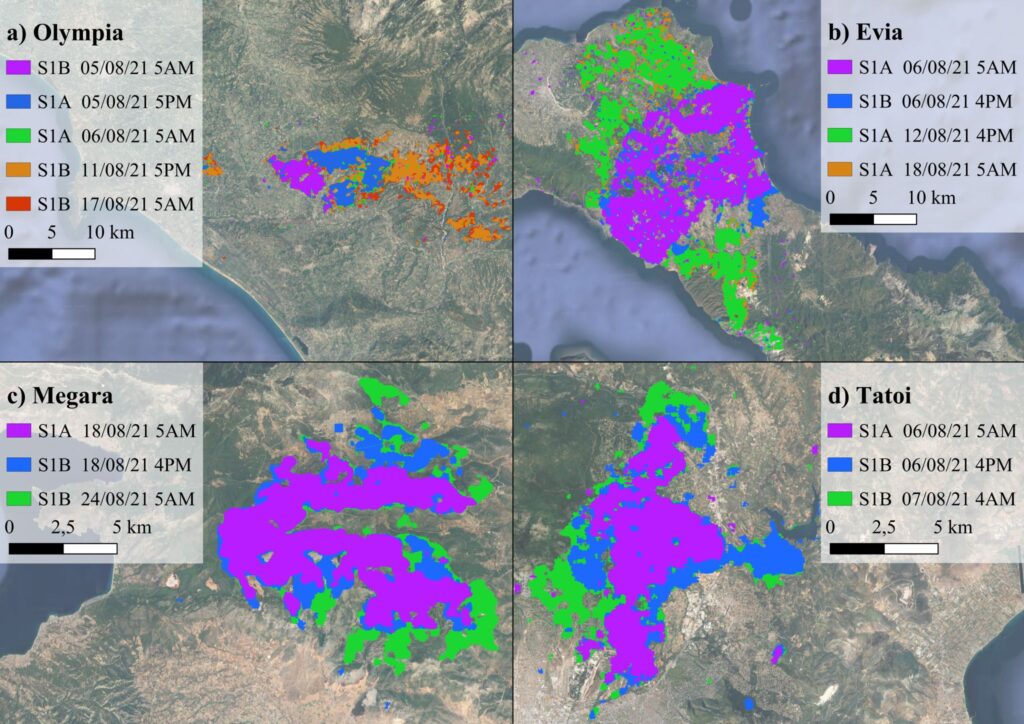
Key takeaways of our study:
🔥 Timely Detection: Sentinel-1 (S1) path overlaps and the availability of both S1 satellites in 2021 allowed us to achieve high temporal resolution, with intervals as short as 12 h between subsequent acquisitions.
⭐ The best results were achieved using both VV and VH features, while a bitemporal ΔRFDI (Radar Forest Degradation Index) and the multitemporal k-map features proved to be the most important ones. Substantial correlations were found between these indices and dNBR from Sentinel-2 optical imagery.
🗺️ Input feature smoothing ensured the right balance between speckle elimination and preserving fine details, while a post-classification filter helped to avoid commission errors.
❗🌱 Challenges were observed in regions characterized by a substantial share of agricultural areas, while burned forests had the highest detection accuracies.
🏔️ Terrain effects revealed no substantial effects on the results.
🌧️ Rainfall events in Evia complicated the detection of burned areas by increasing backscatter values, mimicking unburned surfaces.
🛠️ To make these insights accessible, we developed a GEE Toolbox, called Sentinel-1 Burned Area Progression Toolbox (S1-BAP), a freely available tool to monitor wildfire progression with S1 SAR data, available on GitHub: https://github.com/palubad/S1-BAP
Read the full paper here: https://doi.org/10.1109/JSTARS.2024.3427382
D. Paluba et al., “Tracking Burned Area Progression in an Unsupervised Manner Using Sentinel-1 SAR Data in Google Earth Engine,” in IEEE Journal of Selected Topics in Applied Earth Observations and Remote Sensing, vol. 17, pp. 15612-15634, 2024, doi: 10.1109/JSTARS.2024.3427382.
Abstract:
The frequency of wildfires is increasing worldwide, contributing to a third of forest loss over the last two decades. Tracking burned area progression using traditional optical remote sensing is hindered by cloud and smoke coverage. Therefore, this research employs multitemporal synthetic aperture radar (SAR) satellite data, which are not susceptible to atmospheric effects.
Focusing on four Greek wildfires in 2021, the research utilizes unsupervised k-means clustering on bitemporal and multitemporal SAR polarimetric features. The impact of input feature smoothing with varying moving kernel window sizes was assessed to improve accuracy. The use of these smoothed features led to a substantial improvement in accuracy across all four areas examined, while a window size of 19 × 19 was chosen as the right balance between preserving fine details and minimizing speckle. Furthermore, adding a filter after clustering to remove areas smaller than 2 ha led to additional improvements in accuracy, especially in commission error.
The results using the defined settings revealed F1 scores of 0.75–0.88, overall accuracy of 81%–94%, and omission/commission errors of 33%–16% and 14%–3%, respectively. Challenges were observed in regions characterized by a substantial share of agricultural areas, while terrain effects revealed no substantial effects on the results. The assumption that the SAR will be sensitive mainly to bigger structural changes was proved in the visual validation using high-resolution imagery. In addition, a Google Earth Engine toolbox “Sentinel-1 Burned Area Progression” was developed using the presented methodology and is freely available for the scientific community on GitHub.